Protein Prediction: A real use case for AI and the future of personalized medicine
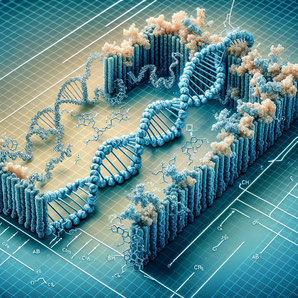
“Humans doing the hard jobs on minimum wage while the robots write poetry and paint is not the future I wanted”, wrote Karl Sharro in a tweet early in 2023 (1). Yet here we are, slowly accepting the results of OpenAIs products creeping into our knowledge hubs. The written word from ChatGPT – no doubt more advanced than Émil Borels monkey – in whose theorem he suggested that if we let a representative of said species type away on a keyboard long enough, eventually something meaningful might emerge (2) – is proofing to be a great partner in crime when it comes to copying form, style and content of the greatest writers from our past. Only the imagination is now left to us human beings, but maybe that’s just a matter of time or maybe complexity in data. While the statistical analysis of all written communication throughout time clearly showcases the abilities of so called AI systems in processing data, the relevance, implications or even dangers to society are yet to be determined.
Therefore, let’s look at a use case that’s not only relevant but whose implications are so widespread that it might be the first step into a new kind of medicine for all, Protein Prediction.
The importance of Protein Prediction
The shape or structure of a protein largely determines how it interacts with other molecules. By predicting its structure, scientists can deduce their functions within the body, how they catalyze chemical reactions, provide structural support, coordinate immune responses, transport molecules and so on. Specific knowledge of these structures is crucial e.g. in the field of drug design, where a lot of research goes into finding out about how they bind to specific proteins and alter their activity. A deeper understanding of this process can lead and actually already has led to more effective and selective drugs with fewer side effects. The advancements of HIV medication and personalized cancer medicine are leading examples of that. (3)
Misfolded proteins are also associated with certain diseases such as Alzheimer’s, Parkinsons or cystic fibrosis where aggregations of such proteins over time become insoluble fibrils that eventually form amyloid plaques – the hallmark features of many such diseases. (4)
But Proteins and their folded states are also crucial in industrial processes, e.g. in the production of biofuels, cleaning agents, food manufacturing and synthetic biology in general. Knowing protein structures allows for the modification of organisms at a molecular level to optimize production.
Challenges in Protein Prediction
- Proteins are made up of amino acids that – due to their complexity – can fold in an almost infinite number of ways. Protein folding is the process by which an unstable condition of a protein becomes a three-dimensional, stable structure that is also biologically functional. Never does this process take place without the inherent risk of mistakes due to interactions with other proteins, DNA, RNA and other smaller molecules.
- The energy landscape that governs these folding pathways are incredibly complex making accurate predictions challenging.
- Compared to the vast number of proteins that can exist, experimental data is still limited, slow and very costly.
- Proteins are not static. They change conformations in response to their environment and capturing these is difficult although crucial for understanding protein function. (5)
AI Innovations: From Anfinsen to AlphaFold
Understanding these complex interactions and their effect on the outcome has its roots already in the 70s when Christian Anfinsen – the nobel laureate for his work on ribonuclease (nobelprize website) – and later Cyrus Levinthal whose rule-based approach to understanding how proteins fold, set the stage for the development of computational methods to predict protein structures. (6)
One of the first approaches that followed their groundwork was the “Homology Model” which relies on the assumption that similar amino acid sequences fold in similar ways and can therefore be used as templates to model unknown structures. Clearly this method had major limits especially when no suitable templates existed, so others such as “Ab Initio Modeling” were developed which are based on physical and biochemical principles with the downside of being computationally intense. Next to early computational models also experimental techniques such as X-ray Chrystallography or Nuclear Magnetic Resonance Spectroscopy were developed. The later – though with less resolution – provides insight into molecules that are difficult to crystallize in general. The latest advancement in the experimental field is the Cryo-Electron Microscopy (or Cryo-EM) which in its current development awarded the Nobel Prize to Jacques Dubochet in 2017. (7)
It was only a year later – in 2018 – when Alphabet Inc. in the name of DeepMind introduced AlphaFold, an AI-driven protein structure prediction tool. It significantly outperformed its competitors in the 13th Critical Assessment of Structure Prediction (CASP13) competition – a biennial event that benchmarks the latest techniques.
AlphaFold was trained on the entire PDB (Protein Data Bank) available at the time, which is a collection of known 3D proteins and other biological molecules. It sources computational models just as much as the results of experimental techniques. Its deep neural network introduced several innovative components such as
- “Attention Mechanism” which allows the model to focus on different parts of the amino acid sequences and how they interact to affect the overall structure of the protein.
- “Spatial Graph Convolutional Networks” that help understanding the spatial relationships and dependencies of amino acids and their side chains in 3D space.
- “End-to-End Differentiability” which is the most crucial part, meaning it can directly predict the distances and angles between amino acids in a protein and therefore predict the entire structure in one go. (8)
AlphaFold 2 has then become widely successful not only due to certain technical upgrades – such as confidence measurements, pairwise distance predictions and utilization of MSA (Multiple Sequence Alignment), but mostly thanks to its release to the public allowing the scientific community to apply the tool to a wide range of challenges in biology, medicine and beyond.
With the mainstream availability of so called AI systems such as ChatGPT, the hardware necessary to compute the unsurmountable amount of data for predicting these protein folding outcomes has become cheaper and more effective as well.
The Impact of AlphaFold on Medicine and Beyond
Now AlphaFold 3 (AF3) has been released, May 8th 2024.
- Unlike AF2, which focused on individual proteins, AF3 embraces complexity of biomolecular interactions. It models diverse entities within a unified framework, highlighting interactions that are crucial for understanding cellular functions and more realistic simulations.
- AF3’s diffusion-based architecture allows more accurate predictions across various molecular types, from ions to modified residues by accommodating arbitrary chemical components without special casing. It also predicts raw atom coordinates directly, unlike AF2.
- The generality of diffusion enables more accurate predictions across various molecular types, from ions to modified residues. Even hallucinations have been reduced in disordered regions using cross-distillation and confidence metrics. (9, 10)
The Future and Limitations of Protein Modeling
- AF3 still can’t properly predict dynamic behavior and has issues managing stereochemical violations.
- While it predicts static structures very well, it can’t capture a full range of conformations in various environments. Therefore its ability to model the full dynamism of nuclear processes remains limited.
- Also, cells compartmentalization is an area that still warrants further research. Interactions with membranes, organelles and varying conditions influence protein behavior massively.
- The current token ceiling of 5000 affects large systems like epigenetic complexes and full nuclear structures. But this will probably increase with better availability of computational power. (11)
The progress from AF2 to AF3 illustrates the rapid evolution of using AI for biological insights. As these models become continuously refined, the potential for breakthroughs in understanding disease and developing new therapies grows exponentially.
All in all the limitations for a more rapid development doesn’t necessarily lie in the AI models themselves, but the fact that most of the proteins themselves and their functions have not yet been characterized. Scientists estimate that 37 % of our proteins are only described rudimentary which still limits the possible outcome of all AI models and protein predictions. (12)
by mario
Bibliography:
(1) https://twitter.com/KarlreMarks/status/1658028017921261569?lang=de (last visit 10.05.2024)
(2) https://demonstrations.wolfram.com/InfiniteMonkeyTheorem/ (last visit 10.05.2024)
(3) https://www.ncbi.nlm.nih.gov/books/NBK26830/ (last visit 10.05.2024)
(4) https://ui.adsabs.harvard.edu/abs/2003Natur.426..900S/abstract (last visit 10.05.2024)
(5) https://rootsofprogress.org/alphafold-protein-folding-explainer (last visit 10.05.2024)
(6) https://www.nobelprize.org/prizes/chemistry/1972/press-release/ (last visit 10.05.2024)
(7) https://bioinformaticsreview.com/20210413/homology-modeling-vs-ab-initio-protein-structure-prediction/ (last visit 10.05.2024)
(8) https://www.nature.com/articles/s41586-021-03819-2 (last visit 10.05.2024)
(9) https://blog.google/technology/ai/google-deepmind-isomorphic-alphafold-3-ai-model/#responsibility (last visit 10.05.2024)
(10) https://www.nature.com/articles/s41586-024-07487-w (last visit 10.05.2024)
(11) https://twitter.com/prmshra/status/1788378945039044743 (last visit 10.05.2024)
(12) https://analyticalsciencejournals.onlinelibrary.wiley.com/doi/10.1002/pmic.201800093 (last visit 10.05.2024)
Background Info:
(13) https://www.youtube.com/watch?v=Mz7Qp73lj9o (last visit 10.05.2024)